Home>Production & Technology>Cover Song>How To Make AI Cover Song
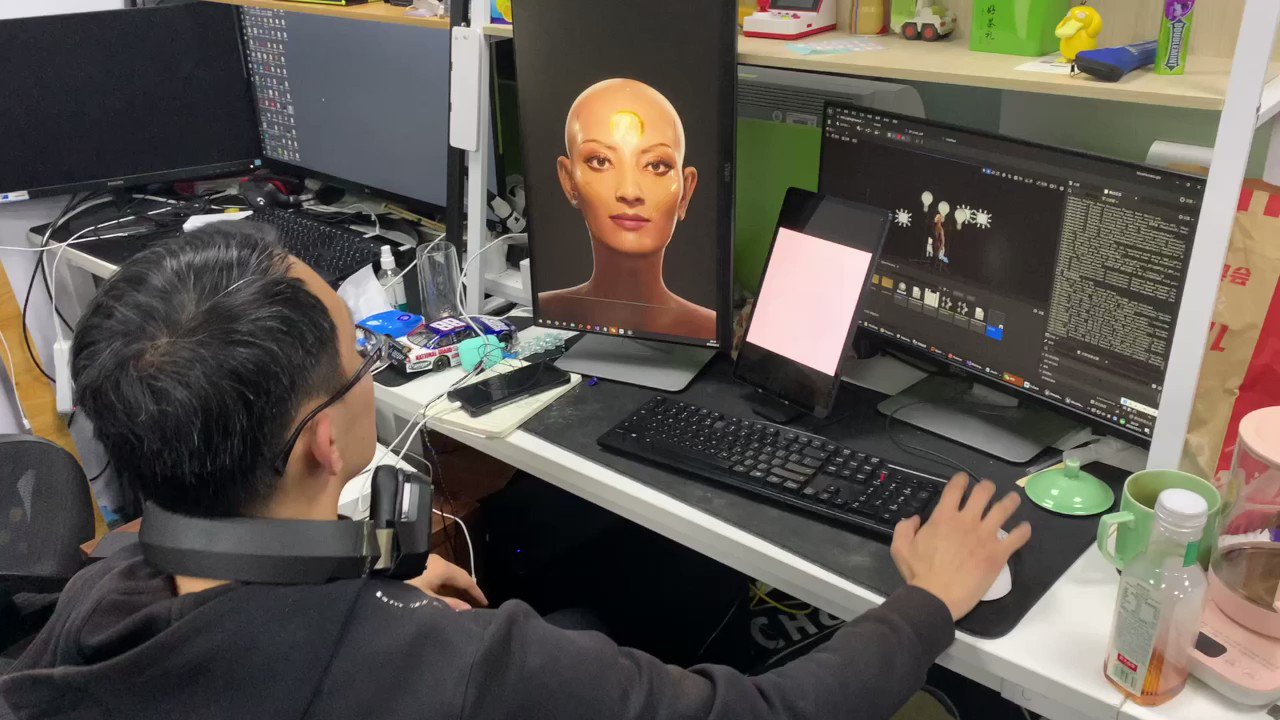
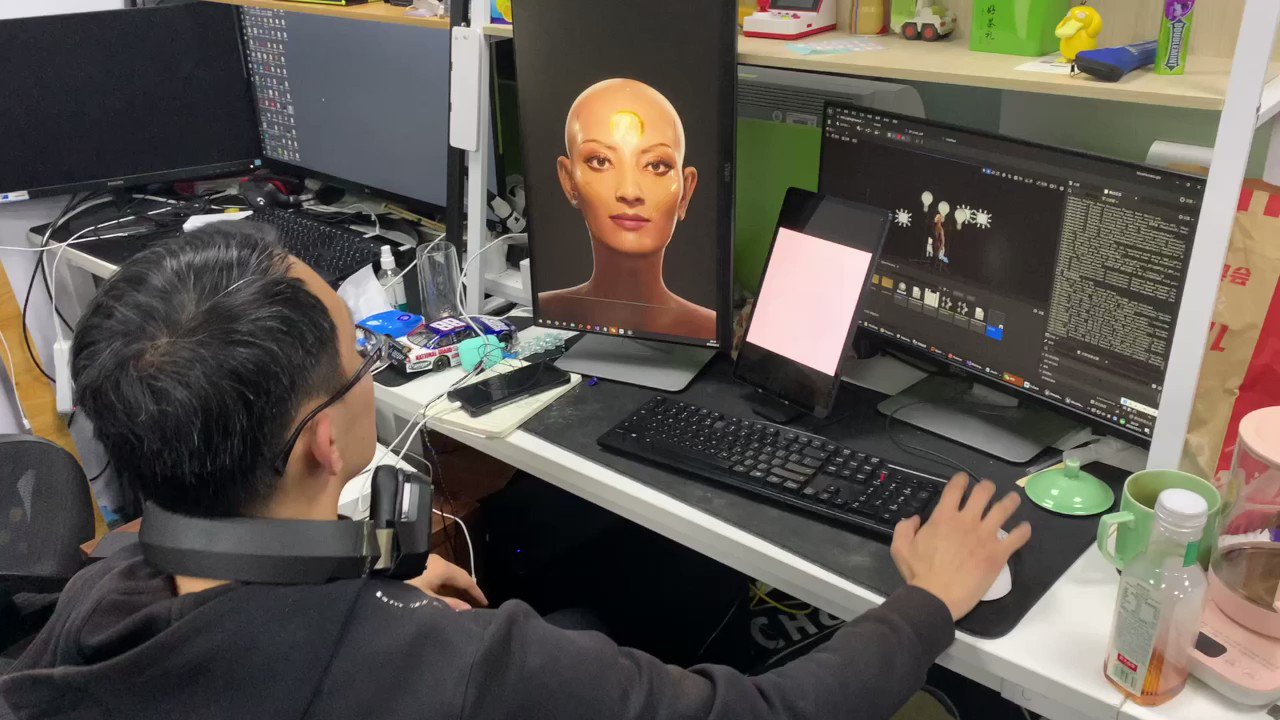
Cover Song
How To Make AI Cover Song
Modified: January 22, 2024
Learn how to create AI-generated cover songs with our step-by-step guide. Turn any song into a unique cover using advanced artificial intelligence technology.
(Many of the links in this article redirect to a specific reviewed product. Your purchase of these products through affiliate links helps to generate commission for AudioLover.com, at no extra cost. Learn more)
Table of Contents
Introduction
Music has always been a universal language that moves us, makes us feel, and brings us together. Among the various forms of musical expression, cover songs have gained immense popularity in recent years. Cover songs are renditions of existing songs performed by different artists, often adding a fresh perspective and interpretation to the original work.
In the age of Artificial Intelligence (AI), the possibilities of creative expression have expanded even further. With AI technology, it is now possible to generate AI cover songs that mimic the styles and sounds of different artists or genres. These AI-generated covers can be both fascinating and entertaining, as they offer a unique blend of familiarity and novelty.
Creating AI cover songs involves training a machine learning model on a vast amount of music data, enabling it to learn the patterns, structures, and characteristics of different genres, artists, and songs. By harnessing the power of AI, musicians and music enthusiasts can explore new avenues of musical exploration and experimentation, pushing the boundaries of creativity.
In this article, we will delve deeper into the world of AI cover songs, exploring the process of collecting data, preparing the dataset, training the AI model, generating the covers, and evaluating their quality. We will also discuss the potential refinements and improvements that can be made to enhance the AI’s ability to create realistic and engaging cover songs.
Whether you are an AI enthusiast, a musician, or simply someone curious about the intersection of technology and music, this article will provide you with valuable insights into the fascinating realm of AI cover songs.
Understanding AI Cover Songs
AI cover songs are generated through the use of machine learning algorithms that analyze patterns and characteristics of existing songs to produce new renditions. These algorithms are trained on large datasets of music, enabling them to learn the nuances and styles of different genres, artists, and songs.
One of the primary techniques used in creating AI cover songs is known as deep learning. Deep learning algorithms, such as neural networks, are designed to mimic the human brain’s ability to process and recognize patterns. By feeding the algorithm with a vast amount of musical data, it can learn to identify and reproduce the unique elements of different songs.
The AI model analyzes various aspects of a song, including melody, chord progressions, rhythm, tempo, and vocals. It breaks down these elements into mathematical representations known as vectors, which are used to generate new musical compositions based on the patterns it has learned.
AI cover songs can be generated in various styles and genres. For instance, the AI model can create a cover song in the style of a specific artist, mimicking their vocal style, instrumentation, and even their songwriting techniques. It can also generate covers in different genres, transforming a pop song into a jazz rendition or a heavy metal track into a classical interpretation.
One of the fascinating aspects of AI cover songs is the ability to blend different styles and create unique combinations. For example, the AI model can generate a cover song that fuses elements of hip-hop and rock, resulting in a dynamic and eclectic musical experience.
However, it’s important to note that AI cover songs are not meant to replace the artistry of human musicians. Instead, they serve as a tool for inspiration and creativity. They can be used as starting points for human musicians to build upon, offering new ideas and possibilities for reinterpretation.
Overall, AI cover songs demonstrate the remarkable capabilities of AI technology in the realm of music. They showcase the potential for machines to understand and reproduce the essence of musical expression, bridging the gap between technology and artistry.
Collecting Data for the AI Model
Collecting a diverse and comprehensive dataset is a crucial step in creating an effective AI model for generating cover songs. The dataset serves as the foundation for the machine learning algorithm to learn and understand the various styles and characteristics of different songs.
There are several approaches to collecting data for the AI model. One common method is to gather a large collection of audio recordings of songs from various genres and artists. These recordings can be sourced from music streaming platforms, online music databases, or even personal music libraries.
It’s important to ensure that the dataset represents a wide range of musical styles, eras, and artists to capture the diversity of music. This helps the AI model to learn the distinct features and patterns associated with different genres and artists.
In addition to audio recordings, other types of data can also be valuable in training the AI model. Lyrics of songs, chord progressions, and metadata such as tempo, key, and time signature can provide important contextual information for the algorithm.
When collecting data for the AI model, it’s essential to consider copyright and licensing restrictions. Ensure that the songs used in the dataset are either in the public domain or obtained with the necessary permissions and licenses to avoid any legal issues.
Curating a high-quality dataset is a time-consuming task that requires careful selection and organization of the songs. It’s also important to preprocess the data, removing any noise or inconsistencies in the audio recordings, and standardizing the format of the metadata to ensure consistency.
Furthermore, it can be beneficial to include a balanced representation of both popular and lesser-known songs in the dataset. This allows the AI model to learn from a broad range of musical styles and discover new and innovative cover song possibilities.
Overall, collecting a diverse and extensive dataset is a crucial step in creating an AI model that can accurately generate cover songs. The quality of the dataset directly affects the AI model’s ability to understand and replicate the unique characteristics of different songs and genres.
Preparing the Dataset
Once the data for the AI model has been collected, it needs to be prepared and formatted to ensure optimal training and performance. Preparing the dataset involves several key steps to enhance the AI model’s ability to learn and generate realistic cover songs.
The first step in preparing the dataset is to clean and preprocess the audio recordings. This includes removing any background noise, normalizing the audio levels, and ensuring consistent quality throughout the dataset. By eliminating any unwanted artifacts or inconsistencies, the AI model can focus on learning the essence of the music itself.
In addition to cleaning the audio recordings, it is also important to standardize the format of the metadata associated with each song. This includes information such as tempo, key, time signature, and even lyrics. A consistent and well-structured metadata format enables the AI model to extract relevant information and utilize it in generating cover songs.
Normalization is another critical step in dataset preparation. This involves transforming the audio recordings and metadata into a standardized range or format. Normalizing the data ensures that the AI model can effectively learn from and compare different songs, regardless of their original variations in volume, tempo, or key.
Splitting the dataset into training, validation, and testing sets is essential to evaluate the performance of the AI model accurately. The training set is used to train the model on a vast majority of the data, while the validation set helps monitor the model’s progress and make adjustments during training. The testing set is used to evaluate the final performance and generalization of the model.
In addition to splitting the dataset, it can be beneficial to apply techniques such as data augmentation to further enhance the AI model’s ability to generalize. Data augmentation involves introducing variations to the audio recordings, such as pitch shifting, time stretching, or adding simulated noise. This helps the AI model to adapt to different musical scenarios and produce more diverse and robust cover songs.
Lastly, it is crucial to ensure that the dataset is well-balanced across genres, eras, and artists. This helps prevent biases and ensures that the AI model can generate cover songs that represent a wide range of musical styles and influences.
Overall, preparing the dataset plays a vital role in the success of the AI model for generating cover songs. Cleaning and normalizing the data, standardizing metadata, splitting the dataset, and incorporating data augmentation techniques all contribute to improving the AI model’s performance, versatility, and ability to generate compelling cover songs.
Training the AI Model
Training the AI model is a crucial step in the process of generating cover songs. It involves feeding the prepared dataset into the machine learning algorithm, allowing it to learn the patterns, structures, and characteristics of the songs.
The training process begins by initializing the AI model with random weights and biases. As the algorithm processes the training data, it adjusts these parameters to minimize the difference between its generated output and the actual target output, using a technique known as backpropagation.
During training, the AI model learns to recognize and extract the underlying patterns and features of the songs from the dataset. It analyzes the audio recordings, metadata, and any additional information provided, such as lyrics or chord progressions.
The duration of the training process can vary depending on factors such as the size of the dataset, complexity of the songs, and computational resources. It typically involves multiple iterations, known as epochs, where the entire dataset is processed by the AI model.
The AI model’s ability to generate realistic cover songs improves gradually throughout the training process. As it continues to analyze and learn from the dataset, it refines its understanding of different musical styles, structures, and nuances.
During training, it is important to monitor the performance of the model using metrics such as loss and accuracy. This helps assess how well the AI model is learning and whether adjustments need to be made, such as fine-tuning the hyperparameters or modifying the architecture of the neural network.
Training the AI model is a computationally intensive task that often requires powerful hardware or access to cloud computing resources. The availability of GPUs (Graphics Processing Units) and specialized deep learning frameworks, such as TensorFlow or PyTorch, can significantly accelerate the training process.
Once the AI model has been trained, it is ready to generate AI cover songs based on the knowledge it has acquired from the dataset. However, it’s important to note that the training process is not a one-time event. The AI model can be retrained or updated with new data to further enhance its capabilities and adapt to evolving music styles and trends.
Overall, training the AI model is a pivotal phase in the generation of cover songs. It allows the AI algorithm to learn and assimilate the characteristics of different songs, paving the way for the creation of unique and compelling AI-generated cover songs.
Testing and Fine-tuning the Model
After training the AI model, it is essential to assess its performance and make any necessary adjustments to optimize the generation of cover songs. This is done through thorough testing and fine-tuning.
Testing the model involves evaluating its ability to generate cover songs that are accurate, coherent, and stylistically aligned with the original songs. It is important to utilize a separate testing dataset that the model has not been previously exposed to. By doing so, we can assess the generalization capabilities of the model.
During testing, various metrics and evaluation criteria can be used to assess the quality of the AI-generated cover songs. These metrics may include measures of melodic accuracy, harmonic consistency, and vocal resemblance, among others. Additionally, human feedback and subjective judgments can provide valuable insights into the perceived quality and authenticity of the covers.
If the model does not produce satisfactory results during testing, fine-tuning is necessary to improve its performance. Fine-tuning can involve adjusting the hyperparameters of the AI algorithm, such as learning rate or regularization, to improve the training process and enhance the model’s capacity to generate realistic covers.
Another approach to fine-tuning is to collect additional data that targets specific areas of improvement. For example, if the model struggles with the accuracy of vocal imitation, the dataset can be expanded to include more nuanced vocal performances for training. This targeted data collection helps the model focus on areas that require refinement.
The fine-tuning process typically involves retraining the model using the updated hyperparameters or additional data. This iterative process helps in iteratively improving the model’s performance and bridging any gaps between the AI-generated covers and the original songs.
Throughout the testing and fine-tuning phase, it is crucial to maintain a feedback loop with human experts, such as musicians or music producers. Their expertise and insights can provide valuable guidance in identifying areas for improvement and ensuring that the AI-generated covers align with the artistic intent and style of the original songs.
Testing and fine-tuning the AI model should be an ongoing process to continuously refine and enhance its capabilities. The feedback and insights gained from testing contribute to the iterative improvement of the model, leading to more accurate, coherent, and engaging AI-generated cover songs.
Generating AI Cover Songs
Once the AI model has been trained and fine-tuned, it is ready to generate AI cover songs. This process utilizes the knowledge and patterns learned from the training dataset to create unique interpretations of existing songs or entirely new compositions.
Generating an AI cover song begins by providing the model with a seed or input, which can be a snippet of a melody, lyrics, or even a description of the desired style or genre. The AI model then applies its learned knowledge to generate a new composition that aligns with the given input.
The AI model analyzes the input and utilizes its understanding of music theory, harmony, rhythm, and stylistic conventions to produce a new rendition. It considers various elements such as melody, chord progressions, instrumentation, and vocals to create a cover song that captures the essence of the given input while incorporating its own unique style.
One of the interesting aspects of generating AI cover songs is the ability to experiment with different input parameters to explore a wide range of possibilities. For example, changing the input seed or adjusting the desired style can result in vastly different cover songs, offering a diverse array of musical interpretations.
The generated AI cover songs provide a fresh perspective on the original songs, offering a blend of familiarity and novelty. They can introduce unique stylistic elements, unexpected chord progressions, or creative variations in instrumentation, resulting in engaging and captivating cover songs.
It’s important to note that while AI cover songs can be impressive and enjoyable, they do not completely replace the artistry and creativity of human musicians. The generated covers serve as a starting point for further exploration and artistic expression.
Moreover, the generation of AI cover songs can inspire and spark new creative ideas for musicians. It can serve as a source of inspiration for reinterpreting songs or exploring new musical directions, expanding the boundaries of musical expression.
Overall, generating AI cover songs is an exciting endeavor that combines the knowledge and patterns learned from training the model with the playful exploration of different inputs and stylistic variations. The AI model’s ability to generate unique renditions adds a fresh dimension to the world of music, offering a new realm of creative possibilities.
Evaluating the AI Covers
Evaluating the AI covers is a crucial step in assessing the quality, authenticity, and overall effectiveness of the generated cover songs. It involves both objective metrics and subjective judgments to determine how well the AI model has succeeded in its musical interpretations.
Objective metrics can include measures such as melodic accuracy, harmonic consistency, tempo consistency, and overall fidelity to the original song. These metrics help determine the technical proficiency of the AI model in reproducing the musical elements of the source material.
Subjective judgments play a vital role in evaluating the emotional impact, artistic expression, and overall appeal of the AI covers. Human experts, including musicians and music producers, can offer valuable insights and feedback on the aesthetic qualities of the covers, assessing how well they capture the essence and intention of the original songs.
Additionally, obtaining feedback from listeners and music enthusiasts plays a significant role in evaluating the AI covers. It is essential to understand their preferences, opinions, and emotional response to the generated covers. Feedback from the audience helps assess the level of engagement, enjoyment, and overall acceptance of the AI covers within the wider music community.
It’s important to keep in mind that the evaluation process should consider the context and purpose of the AI model. If the goal is to faithfully replicate a specific artist’s style, the evaluation should focus on the accuracy of the imitation. However, if the aim is to explore innovative and creative reinterpretations, the evaluation may prioritize originality and artistic expression.
Continuous iteration and refinement based on evaluation feedback are crucial for improving the quality and authenticity of the AI covers. The feedback obtained from experts and listeners plays an integral role in identifying areas for improvement, recalibrating the AI model, and guiding future training and fine-tuning steps.
It is important to note that AI covers should be seen as a complement to human creativity rather than a replacement. The goal is to enhance musical exploration, encourage collaboration, and inspire new artistic directions by leveraging the capabilities of AI technology.
Overall, by employing a combination of objective metrics, expert judgments, and audience feedback, the evaluation of AI covers provides valuable insights into the success of the generated cover songs, guides future enhancements, and ensures the continued evolution of AI-generated music in a manner that resonates with human listeners.
Refining and Improving the Model
Refining and improving the AI model is an ongoing process that involves continuous iterations and enhancements to enhance its capabilities in generating high-quality and compelling cover songs.
One approach to refining the model is to gather additional data that targets specific areas for improvement. This can include collecting more diverse songs, introducing variations in musical styles, or obtaining data with more nuanced performances. The inclusion of targeted data helps the model to improve its understanding and expression of different musical elements.
Refining the model also involves fine-tuning the hyperparameters and architecture of the AI algorithm. Adjusting parameters such as learning rate, regularization, or the number of layers can improve the model’s performance and its ability to generate more coherent and stylistically accurate AI covers.
Moreover, incorporating advancements in machine learning and AI technologies can enhance the model’s performance. Stay up-to-date with the latest research and techniques in the field, as new methods and approaches may yield improvements in the generation of cover songs. Experimenting with different algorithms and frameworks can also offer insights into optimizing the model’s performance.
Feedback from human experts, musicians, and listeners plays a crucial role in refining the AI model. Actively seek input and opinions to gain insights into areas that require improvement or further development. By incorporating this feedback, the model can better align with artistic intent and produce more authentic and enjoyable AI covers.
Regular evaluation and testing of the AI covers are essential to monitor the model’s performance and identify areas for refinement. Analyze the strengths and weaknesses of the generated covers and use this knowledge to guide future iterations and enhancements of the model.
Collaboration with other researchers and AI enthusiasts can also contribute to refining and improving the AI model. Engage in discussions, attend conferences and workshops, and share knowledge with the community to stay informed about the latest techniques and approaches in generating AI covers.
It’s also important to acknowledge and address ethical considerations when refining the AI model. Ensure that the model respects copyright and intellectual property rights, and that the generated covers do not infringe upon any legal limitations or restrictions.
Ultimately, the process of refining and improving the AI model is an iterative and ongoing one. Embrace a growth mindset and recognize that continuous learning, experimentation, and incorporation of feedback are key to developing a robust and versatile AI model for generating high-quality and captivating cover songs.
Conclusion
The world of AI cover songs offers a fascinating intersection of technology and music. Through the use of machine learning algorithms and deep neural networks, AI models are capable of analyzing patterns and characteristics in music to generate unique and captivating cover songs.
Throughout this article, we explored the process of creating AI cover songs, starting with collecting a diverse dataset that represents various genres, artists, and musical styles. We then discussed the importance of preparing the dataset, including cleaning the audio recordings and standardizing metadata.
The training phase of the AI model involves fine-tuning its parameters and allowing it to learn from the dataset, while the testing phase evaluates its performance and generalization capabilities. We also explored the process of generating AI cover songs, where the model utilizes its learned knowledge to create novel interpretations of existing songs or entirely new compositions.
Evaluation of the AI covers combines both objective metrics and subjective judgments to assess their quality, authenticity, and artistic appeal. The feedback obtained from experts and listeners plays a crucial role in refining and improving the AI model and ensuring its alignment with human artistic expression.
It is vital to recognize that AI cover songs are not meant to replace human creativity but rather to inspire and offer new possibilities for musical exploration and interpretation. The collaborative efforts between AI models and human musicians can lead to exciting and innovative musical experiences.
As technology continues to advance, refining the AI model through continuous iteration, incorporating additional data, and embracing advancements in machine learning techniques will further enhance the generation of AI cover songs. The ongoing collaboration, feedback, and evaluation from experts and listeners will contribute to the evolution and progress of this creative field.
In conclusion, AI cover songs represent a remarkable fusion of technology, artistry, and musical expression. They open new doors for creativity, artistic collaboration, and inspire musicians to explore uncharted territories. With the power of AI, the world of cover songs becomes a limitless canvas for innovation and reimagination, enriching the musical landscape for years to come.